It’s been nearly a decade since Rajeev Rastogi – Vice President, Machine Learning joined Amazon India. Over the years, he has led and scaled the India Machine Learning (IML) team massively. Last year, the IML team launched 44 models into production, providing a huge boost in revenues. We spoke to him to understand more about Machine Learning, how it impacts Amazon’s business, his journey here, and his favorite Leadership Principle.
Rajeev, please help us understand what Machine Learning is.
ML systems discover hidden patterns in data and leverage those patterns to make predictions about future data. This is very similar to how children learn a new language or learn to recognize objects. For example, a five-year-old child can speak grammatically correct English without knowing a single rule of grammar. Essentially, the child learns to speak the language from listening to adults speaking around him/her/them. Similarly, ML systems learn from examples.
Is there a way for Amazonians who don’t have experience in ML to move into this field?
We have an internal program called Machine Learning Gurukul which helps a Software Development Engineer (SDE) transition to an Applied Science role within 18 to 30 months. There are all kinds of tutorials available on advanced ML topics, apart from mentoring, and on-the-job projects. So far, 30+ SDEs have enrolled and 5 have already become applied scientists. In addition, we have programs that help SDEs get exposure to building ML models. Through ML Apprentice, SDEs get to work on ML problems that are not very complex and deploy models into production with guidance from an applied scientist. That’s a good program for SDEs to get their feet wet and get a flavor of what’s involved in building a model.
What are some of your proudest achievements in your time here at Amazon?
I am super proud of the world class ML team we’ve built here and the business impact the team has been delivering for Amazon in India – it ranges in the billions. I am prouder still of the India-first innovations that’ve emerged out of the IML team. A couple of them are SEAL (Science of Entity Abuse Localization) experts, which helps to pinpoint the entity responsible for a missing item in an Amazon package’s journey – it could be a seller, delivery associate, or customer. There’s also Nostradamus, a technology that mines millions of customer reviews to help us understand what features or products customers care most about; this allows us to give customers exactly what they want.
IML has also published research papers both internally and externally; as a scientist that makes me very proud. This year alone, we had 26 accepted papers at the Amazon Machine Learning Conference. That’s a remarkable number. Seven of these papers were oral presentations, which are very hard to get.
Tell us about your favorite Leadership Principle, along with an example of how you applied it.
I am passionate about innovation, so my favorite Leadership Principle is ‘Think Big’. This LP is about creating a bold vision for the future that is bigger than the current reality – like Prime Air’s vision to deliver packages to customers within 30 minutes. Think Big encourages making bold bets by pursuing radical new approaches with long-term focus, and looking around corners to serve customers better.
This LP helped us incubate several seed initiatives in the India ML org. These are early exploratory bets with high science risk but have the potential to grow into substantial programs in the future. I am particularly excited about these two bets:
- Produce Grading : The quality of produce, i.e. fruits and vegetables, is the single most important customer input in the grocery business and the number one driver of repeat purchase. The current manual assessment of quality is error-prone and costly, not scalable, and has low repeatability. We developed a computer-vision system for grading fresh produce quality by analyzing images to detect defects such as cuts, cracks, pressure damage, etc. This, along with an invention called Autograder that grades produce moving on a conveyor belt, can reduce grading costs by 78%.
- Conversational Shopping Assistant : The vision for this innovation is to provide online shoppers with a conversational shopping experience much like an offline store assistant. This is very important for our next 500 million customers, who will come from smaller towns and villages. They are used to having conversations with the shopkeeper or store assistant to decide which product they should buy. The virtual shopping assistant will give customers a similar experience and guide them to the product that meets their needs.
What does Day 1 mean to you?
Day 1 means three things to me:
- Prioritizing customer experience over short-term profit :
This involves relentless focus on protecting and improving the customer experience. For example, when it comes to pricing, we keep our prices low to earn customer trust in the long run. We do this even when there may be opportunities for us to charge higher prices and increase our short-term profit (like when a product is in short supply). We may make less per item, but by consistently earning trust we will sell many more items. - Making bold bets with long-term focus :
This involves taking risks and experimenting a lot – you cannot have innovation without a culture of experimentation. Marketplace is a great example of a bold bet. It allows third party sellers to list products on our product detail page and compete with our retail business. Many people were worried that it would cannibalize our retail business, but we became more attractive to customers because we increased unique selections. This in turn drew in more sellers, which got us economies of scale, allowing us to lower prices and eliminate shipping fees for qualifying orders. High-velocity decision making :
This involves making two-way door decisions quickly, while deliberating more on one-way door decisions. We want to be quick in making two-way door decisions since these are easy to reverse. Examples include choosing the image size on the product detail page.On the other hand, one-way door decisions that are not easily reversible need debate, data analysis, and a great deal of deliberation. For example, should we increase Prime subscription fees, or should we charge a fee for customers returning a product.
That’s enough work talk. Tell us more about your love for travel!
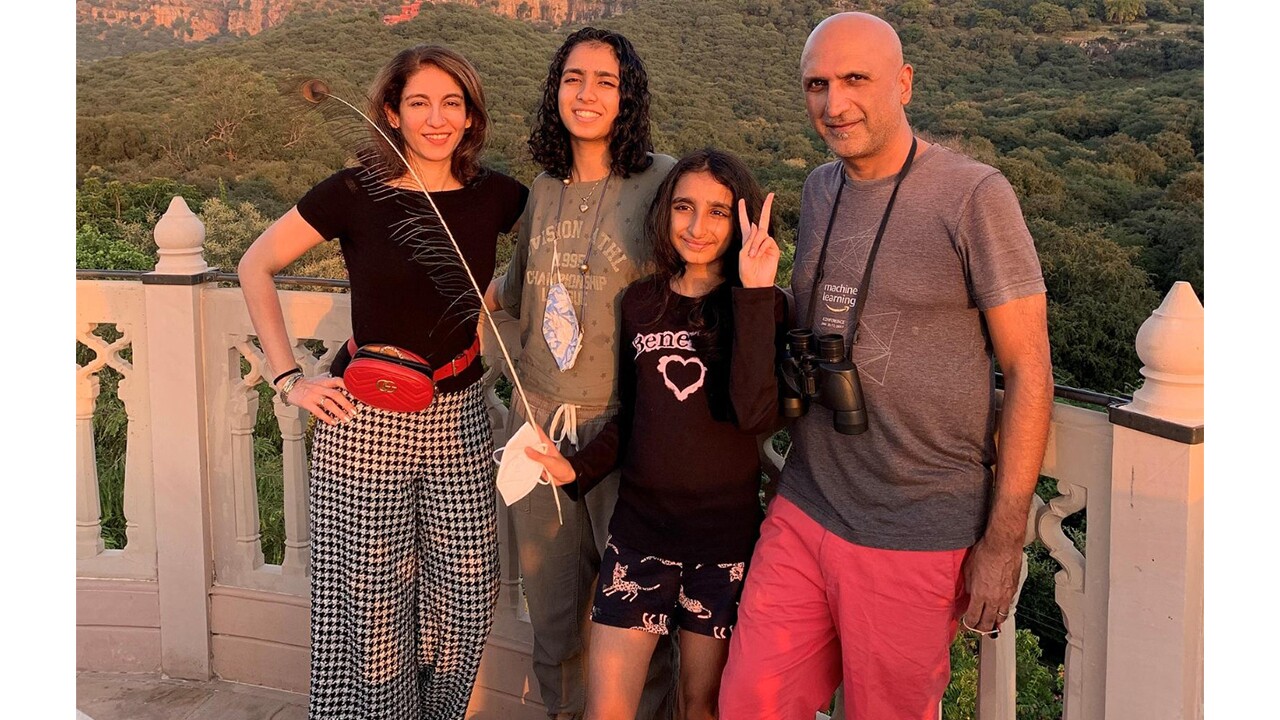
Being a researcher has given me opportunities to see quite a bit of the world. I’ve traveled to 30+ countries and look forward to visiting more, but Goa is by far my number one holiday destination. It’s the place I’ve visited the most.
My relationship with Goa began over 35 years ago when I was a student. Since then I’ve visited the state numerous times. The minute I land in Goa, I switch to vacation mode. There’s something in the air there that makes you feel relaxed and forget about all your problems! And then there’s the tropical weather, the greenery, the warm sea, the beach shacks serving amazing food, the vibrant nightlife, the luxury hotels and villas… I have very fond memories of Goa.